Top 6 Deep Learning Applications
Deep learning has emerged as a transformative technology within the field of artificial intelligence (AI). With its ability to automatically learn and extract meaningful patterns and representations from complex data, deep learning has revolutionized various domains, ranging from computer vision and natural language processing to healthcare and autonomous driving.
In this article, we will explore the key applications in deep learning.
1. Understanding Deep Learning
Deep learning is a subset of machine learning that focuses on training artificial neural networks with multiple layers to analyze and interpret data. Unlike traditional machine learning algorithms, which rely on handcrafted features, deep learning algorithms learn hierarchical representations directly from raw data.
This ability to automatically learn hierarchical representations makes deep learning models capable of capturing intricate relationships and extracting high-level features.
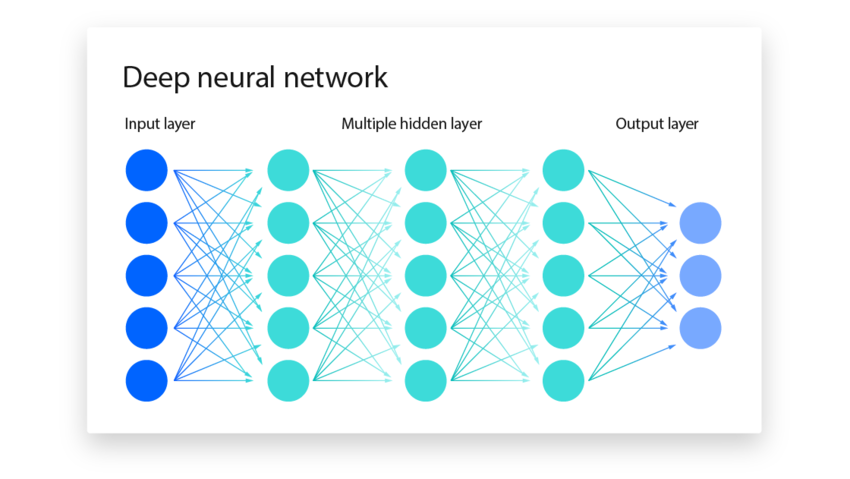
2. Deep Learning Applications
2.1 Deep Learning and Computer Vision
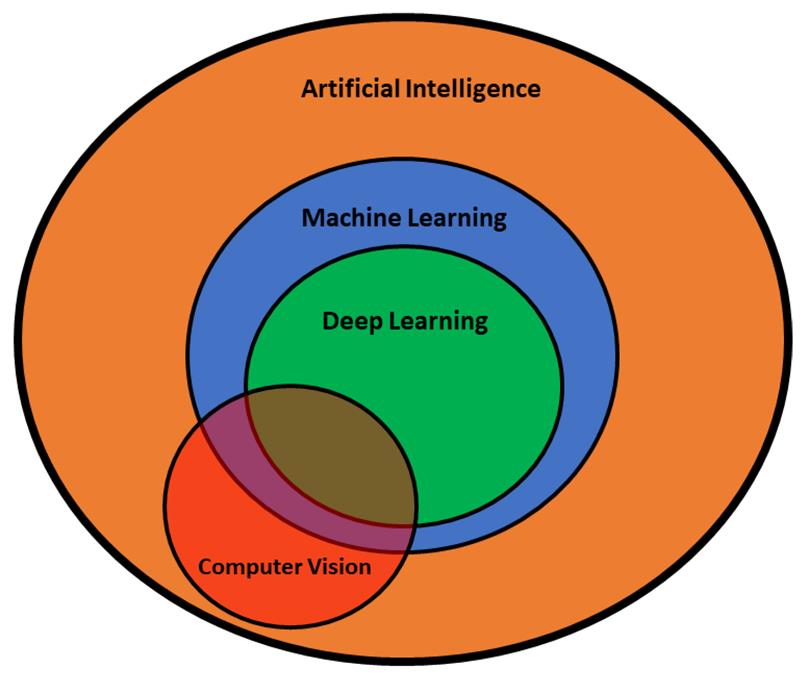
Deep learning has made significant advancements in the field of computer vision, which involves teaching machines to interpret and understand visual information such as images and videos. Deep learning algorithms have revolutionized computer vision tasks like object detection, image classification, and image segmentation.
- Object detection
Deep learning algorithms can be trained to detect specific objects within an image, such as people or objects. This technology is crucial for applications like autonomous driving, where vehicles need to detect and respond to different objects in their environment. It is also used in robotics and healthcare to analyze medical images and identify anomalies or abnormalities.
- Image classification
Deep learning models can classify images into different categories or labels. For example, they can classify images of animals into specific species or categorize images based on their content. Image classification is used in a wide range of applications, including content filtering, visual search, and automated image tagging.
- Image segmentation
Deep learning algorithms can segment an image into different regions or objects. This technique is particularly useful in tasks where precise localization and identification of objects are required. For instance, in medical imaging, deep learning can segment organs or tumors from scans, aiding in diagnosis and treatment planning.
2.2 Deep Learning and Natural Language Processing (NLP)
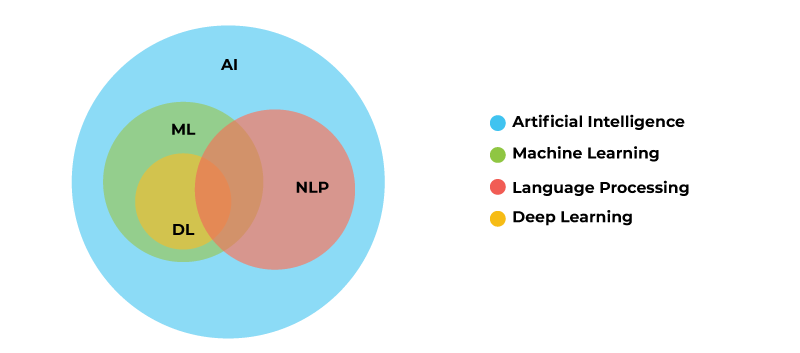
Natural Language Processing (NLP) focuses on the interaction between human language and computers. Deep learning has significantly advanced the field of NLP by enabling computers to interpret and respond to human language in a more sophisticated manner.
- Machine translation
Deep learning models have been successfully trained on large datasets of human language to accurately translate text from one language to another. This technology has widespread applications, including website localization, document translation, and language learning tools. Deep learning-based machine translation systems have significantly improved translation quality and accuracy compared to traditional rule-based approaches.
- Sentiment analysis
Deep learning algorithms can analyze text to determine the emotional tone or sentiment expressed by the author. Sentiment analysis involves classifying text as positive, negative, or neutral. This capability is valuable for businesses to gain insights into customer opinions and feedback on their products or services. It allows companies to make data-driven decisions, enhance customer satisfaction, and improve their offerings.
- Language generation
Deep learning models can generate human-like text, such as chatbot responses or automated content generation. These models are trained on large text corpora and can generate coherent and contextually relevant responses. Language generation has applications in chatbots, virtual assistants, content creation, and personalized messaging.
- Question-answering systems
Deep learning techniques have also been applied to develop question-answering systems that can understand and respond accurately to user queries. These systems can process and analyze large amounts of textual data, extract relevant information, and provide precise answers to user questions.
2.3 Deep Learning and Healthcare
Deep learning has had a significant impact on the field of healthcare, particularly in medical imaging and personalized medicine. It has the potential to improve diagnostics, treatment planning, and patient outcomes.
- Medical imaging
Deep learning algorithms can analyze medical images, such as X-rays, CT scans, and MRIs, to detect abnormalities and assist in diagnosis. These algorithms can learn from vast amounts of labeled medical image data to identify patterns and features that may not be easily visible to the human eye.
For example, deep learning models can detect early signs of cancer in mammograms, identify blood clots in brain scans, and diagnose degenerative diseases in spinal cord images. By providing more accurate and timely diagnoses, deep learning enhances the efficiency of healthcare delivery and improves patient care.
- Personalized medicine
Deep learning algorithms can analyze large datasets of patient information, including genetic data, medical records, and treatment outcomes, to identify patterns and correlations. This information can be used to develop personalized treatment plans tailored to an individual's genetic makeup and specific medical conditions.
Deep learning-based personalized medicine has the potential to optimize treatment efficacy, minimize side effects, and improve patient outcomes.
- Drug discovery
Deep learning techniques are also being applied to accelerate the drug discovery process. By analyzing vast amounts of chemical and biological data, deep learning models can predict the effectiveness of potential drug candidates, identify promising drug targets, and help researchers prioritize their efforts. This can significantly reduce the time and cost involved in developing new drugs and therapies.
2.4 Deep Learning and Finance
Deep learning techniques have found valuable applications in the financial industry. They contribute to fraud detection, risk management, and enhancing the overall customer experience.
- Fraud detection
Deep learning algorithms can analyze vast amounts of financial data to identify patterns and anomalies that may indicate fraudulent activities. By learning from historical data, these models can detect suspicious transactions, unauthorized access, or fraudulent behaviors, helping financial institutions prevent and mitigate fraud.
- Risk management
Deep learning models can assist in assessing and managing financial risks. They can analyze complex datasets and identify patterns that may indicate potential risks, such as market volatility or credit default. These models enable financial institutions to make more accurate predictions and informed decisions in areas like investment management, loan underwriting, and portfolio optimization.
- Customer experience
Deep learning techniques can personalize financial services and improve the customer experience. By analyzing customer data, transaction histories, and preferences, deep learning models can provide personalized recommendations, tailored financial advice, and customized product offerings. This personalization enhances customer engagement, satisfaction, and loyalty.
2.5 Deep Learning and Agriculture
Deep learning has the potential to revolutionize the agricultural industry by optimizing crop monitoring, resource usage, and yield prediction. By leveraging data from various sources, such as satellite and drone imagery, weather data, and soil sensors, deep learning models can improve crop health and productivity.
- Crop monitoring
Deep learning algorithms can analyze satellite or drone images of fields to monitor crop health and identify areas of concern. These models can detect disease outbreaks, nutrient deficiencies, or pest infestations at an early stage, enabling farmers to take proactive measures to protect their crops.
- Resource usage optimization
Deep learning models can analyze data from soil sensors, weather forecasts, and historical crop information to optimize resource usage. They can determine the optimal timing and amount of irrigation, fertilization, and pesticide application based on specific crop needs, weather conditions, and soil characteristics. This helps farmers maximize yield while minimizing resource waste and environmental impact.
- Yield prediction
Deep learning algorithms can predict crop yields based on historical data, weather patterns, and other relevant factors. Accurate yield predictions enable farmers to make informed decisions regarding planting schedules, crop rotation, and marketing strategies. This information helps optimize production, supply chain management, and financial planning.
2.6 Deep Learning and Cybersecurity
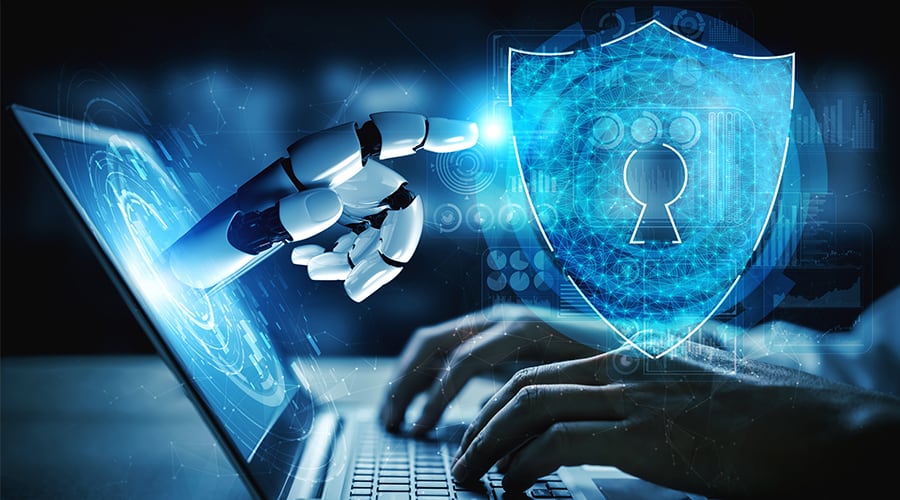
Deep learning techniques play a vital role in cybersecurity by aiding in threat detection, malware detection, and user behavior analysis. They help identify security breaches, protect systems and networks, and ensure data privacy.
- Threat detection
Deep learning models can analyze network traffic, system logs, and other security data to detect and respond to potential threats. By learning from historical patterns and anomalies, these models can identify suspicious activities, such as unauthorized access attempts or abnormal network behavior, enabling timely intervention and mitigation.
- Malware detection
Deep learning algorithms can analyze large volumes of data, including files, emails, and network traffic, to detect and classify malware. These models can learn to identify patterns and characteristics of malicious software, even in previously unseen or evolving malware variants. Deep learning-based malware detection systems help protect against emerging cyber threats and enhance overall system security.
- User behavior analysis
Deep learning models can analyze user behavior patterns and detect anomalies that may indicate unauthorized access or malicious activities. By monitoring user actions, access logs, and system interactions, these models can identify deviations from normal behavior and raise alerts for potential security breaches.
Conclusion
The applications of deep learning are vast and diverse, spanning various industries and domains. From healthcare to marketing, finance to agriculture, and beyond, deep learning is transforming how we solve complex problems, make predictions, and extract insights from data.
As technology advances and datasets grow, deep learning is expected to continue driving innovation and pushing the boundaries of artificial intelligence.